YOGUSENSE
SUMMARY
YOGUSENSE aims to capitalize on the capabilities of softsensing to mainstream the application of process analytical technology among small-medium food producers. The project will demonstrate the commercial feasibility as well as economic and environmental benefits of a platform fusing data from inline low-cost sensors, offline analyzers, and other process data to create softsensors and optimization models for in-process and real- time quality control in a fermentation-based process (Strained yogurt production).
DESCRIPTION
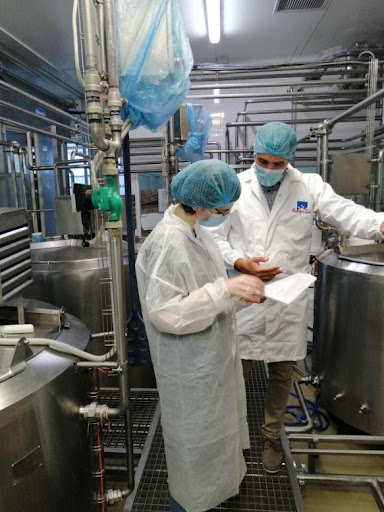
Process Analytical Technology (PAT) is a system (including instruments, methods and strategies) for designing, analyzing and controlling manufacturing processes through measurements of critical process parameters (CPP) and Critical Quality Attributes (CQA) of raw and processed materials to improve product quality, increase efficiency and reduce costs. PAT has effectively been employed for several years, mostly in pharmaceutical and other high margin industries (e.g. chemicals), bringing about important economic and environmental benefits while increasing the competitiveness of those industries.
Although it is proven that food industries could reap similar benefits with pharma from the implementation of PAT, the complex nature of the biological processes involved, such as fermentation, the strict legislations regarding their operating conditions (food grade) and the high CAPEX of complex inline sensors (e.g. laser or hyperspectral) makes PAT application technically complex, time-consuming and, ultimately, too expensive for an industry with low margins and with a very strong presence of SMEs who lack the capital for investing in such high-end sensing and automation systems. At present, the variables which can be automatically detected mainly center on variables such as temperature, tank pressure, pH value, dissolved oxygen, stirring speed and so on. Key variables which have a critical influence on productivity and quality such as cell concentration, substrate concentration, and product concentration cannot be measured with practical online measuring instruments or they require expensive custom-made instrumentation. Offline testing has a time-lag problem, and cannot satisfy the needs of real-time optimization control.
In recent years, advances in data analytics have given rise to the concept of the Softsensors which offers a solution to the above problems, allowing food industries to benefit from the advantages of PAT at lower costs. By using historical data series including both CPPs and CQAs, it is possible to detect correlations between different CPPs and CQAs and infer the values of the latter from combinations of simple measurements that do not require expensive sensors or complicated installations to be measured. This allows for the radical reduction of PAT application costs while maintaining most benefits.
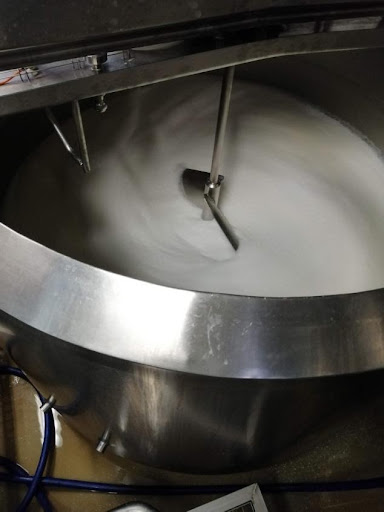
YOGUSENSE aims to capitalize on the capabilities of softsensing to address these problems and mainstream the application of PAT among small-medium food producers by demonstrating in a fermentation-based process (Strained yogurt production) the commercial feasibility as well as economic and environmental benefits of a PAT platform fusing data from inline low-cost sensors, offline analyzers, and other process data to create softsensors and optimization models for in-process and real-time quality control. All these are encapsulated in a digital solution to facilitate data management, application of predictive models and decision support to operators.
The application aims to prove that with targeted interventions in key steps of the yogurt production process, even dated production lines can be economically retrofitted and upgraded to provide high quality products. To achieve this, YOGUSENSE brings together a consortium of 2 SMEs specialising in the development of sensing applications (Castalia and IQ of Things) and an end-user (Mandrekas Dairy Products S.A.).
YOGUSENSE has received funding from the European Union’s Horizon 2020 research and innovation programme within the framework of the S3FOOD project, funded by the European Union’s Horizon 2020 research and innovation programme under grant agreement no. 824769.